- 課程時間
- 課程簡介
- 課程特點
- 認證要求
- 考試須知
- 課程內容
傳統服務:課程上堂時間表 (地點:旺角 總費用:$8,940) 學員使用電話或本網頁報名,待本中心確認已為學員留位後,即可使用 繳付學費,過程簡便!
超震撼: |
凡於 2024年 9月 13日 (五) 或之前報讀本課程, 原價 $11,175,現只需
$8,940! |
|
* 各政府部門可使用 P Card 付款
如使用 P Card 繳付考試費,考試費需另加 1.3% 附加費
*** 質素保證:
免費於任何地點試睇首 3 小時課堂錄影,從而可預先了解導師及教材的質素,才報讀課程來上堂。***
請致電與本中心職員預約。
查看各地點電話
旺角 |
2332-6544 |
觀塘 |
3563-8425 |
北角 |
3580-1893 |
沙田 |
2151-9360 |
屯門 |
3523-1560 |
免費補堂: |
學員可於任何地點補看課堂錄影,從而可銜接往後的課堂! |
免費重讀: |
學員可於課程結束後三個月內於任何地點不限次數地重看課堂錄影,從而可反覆重溫整個課程! |
課時: |
42 小時 |
課堂導師: |
Larry (任教課程清單) |
|
注意! 客戶必須查問報讀學校的教育局註冊編號,以確認該校為註冊學校,以免蒙受不必要的損失!
本中心是 Microsoft 認可的合作夥伴
(Microsoft Certified Partner)。
|
人工智能是什麼?
人工智能 (AI) 是展現一種或多種接近人類能力的軟體:從視覺感知到分析學習、解決問題和作出決策等等。
人工智能 (AI) 在我們日常使用的軟體應用程式中越來越普遍;包括我們家裡和手機中的數位助理、車輛中的自動駕駛技術,以及幫助我們完成工作的生產力應用程式。
透過 AI,機器可以分析資料、文字和影像、理解語音、以自然方式互動,以及使用資料進行預測,例如電腦何時需要維護,或客戶可能想要購買何種類型的產品。
Azure 是彈性的平台,可讓您的組織充份利用 Microsoft AI 及 Open AI 服務。當您使用以 Azure 雲端為運行基礎的新一代智慧型應用程式時,您仍然可以自行決定放置企業資料的位置,例如雲端 (cloud)、內部部署 (on-premises),以及智慧邊緣 (intelligent edge)。
Azure 能讓您擁有:
- 視覺感知 - 使用電腦視覺功能來處理影像、視訊串流和即時攝影機輸入影片。
- 文字分析與對話 - 使用自然語言處理 (Natural Language Processing & Large Language Models) 不僅可以閱讀,還可以產生真實的回應並從文件中提取要點及作出總結的能力。
- 語音 - 辨識語音輸入並合成語音輸出的能力。語音功能與自然語言處理分析的能力相結合,實現了一種被稱為對話式 AI 的人機交談形式。
- 作出決策 - 利用過去的經驗和學習 (Machine Learning) 來評估情況並採取適當行動的能力。例如,識別感應器不尋常讀數 (Multivariate Multiple Regression) 並採取自動化回應操作來防止故障或系統損壞。
- Microsoft 及 Open AI 的人工智能技術。
- 最完整的合規性和安全性產品。
AI 應用程式是融合了 AI 功能的 Web 或行動應用程式,例如視覺辨識或語言處理。具體例子如使用臉部辨識作為行動裝置登入程序一部份的金融服務應用程式,就是融合了 AI 功能的行動應用程式。
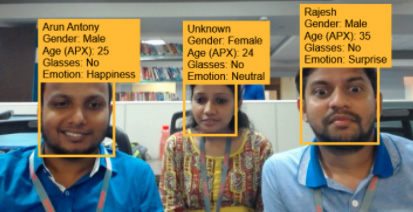
AI 代理程式則是使用 AI 功能與真人使用者互動的電腦程式。例如,某公司使用聊天 Bot 管理即時的 Web 式客戶服務要求,即為 AI 代理程式的範例。
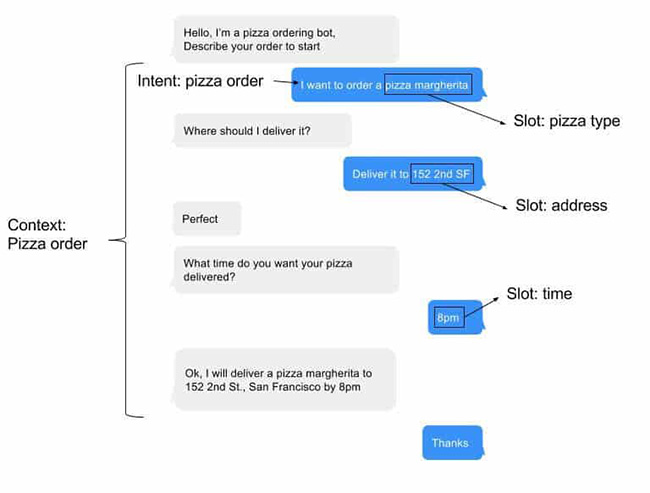
透過租用或購買 Microsoft 的智慧型應用程式和代理程式的 AI 模型 (Pre-trained models),令您可以親自制定您的商業應用程式來提升您的產品及服務水平!
Microsoft 利用 Azure 認知服務讓您的應用程式有突破性體驗。各種產業以及各種不同的產品,例如 Microsoft 365、Xbox 與 Bing,現在都使用領先的 AI 模型。
關於 AI Engineer 認證
Azure AI Engineer Associate 認證的應試者應具有機器學習 (ML) 和人工智慧 (AI) 概念,以及相關 Microsoft Azure 服務的實務知識。這認證是一個展示常見 AI 工作負載以及如何在 Azure 上實際運作 AI 技巧的機會。
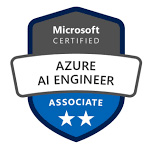
Microsoft Certified: Azure AI Engineer Associate
About the course
通過本課程,您將學習管理、部署和利用 Azure AI 認知服務 (Cognitive services)、AI 搜尋 (Search and Indexing),並了解如何使用基於 REST 的 API 在 Azure 上建立電腦視覺、語言分析、知識挖掘、智慧搜尋和 Open AI 的生成式解決方案。
我們的資深講師 Larry Chan 將為您提供各種 Microsoft Azure 認知服務 (Cognitive services) 及 Open AI 相關產品的建議和運用技巧。
|
課程名稱: |
Microsoft Certified Azure AI Engineer Associate (1 科 Microsoft 雲端人工智能) 國際認可證書課程 - 簡稱:Azure AI Engineer Training Course |
課程時數: |
42 小時 (共 14 堂,共 1 科) |
適合人士: |
有志考取 Microsoft Certified: Azure AI Engineer Associate 證書人士 |
授課語言: |
以廣東話為主,輔以英語 |
課程筆記: |
本中心導師親自編寫英文為主筆記,而部份英文字附有中文對照。 |
1. 模擬考試題目: |
本中心為學員提供模擬考試題目,每條考試題目均附有標準答案。 |
2. 時數適中: |
本中心的 Microsoft Certified Azure AI Engineer Associate (1 科 Microsoft 雲端人工智能) 國際認可證書課程時數適中,有 42 小時。
令學員能真正了解及掌握課程內容,而又能於 3 個月內考獲以下 1 張國際認可證書:
- Microsoft Certified Azure AI Engineer Associate
|
3. 導師親自編寫筆記: |
由本中心已擁有五項 MCITP , 十多項 MCTS,MCSA 及 MCSE 資格,並有教授 Microsoft 相關課程
24年以上經驗的資深導師 Larry Chan 親自編寫筆記,絕對適合考試及實際管理之用,令你無須「死鋤」如字典般厚及不適合香港讀書格調的書本。 |
4. 一人一機上課: |
本課程以一人一機模式上課。 |
5. 免費重讀: |
傳統課堂學員可於課程結束後三個月內免費重看課堂錄影。 |
Microsoft 已公佈考生只要通過以下 1 個 Azure AI 相關科目的考試,便可獲發 Microsoft Certified Azure AI Engineer Associate 國際認可證書:
考試編號 |
科目名稱 |
AI-102 |
Designing and Implementing a Microsoft Azure AI Solution |
本中心為Microsoft指定的考試試場。報考時請致電本中心,登記欲報考之科目考試編號、考試日期及時間
(最快可即日報考)。臨考試前要出示身份證及繳付每科HK$1,025之考試費。
考試題目由澳洲考試中心傳送到你要應考的電腦,考試時以電腦作答。所有考試題目均為英文,而大多數的考試題目為單項及多項選擇題,其餘則為實戰題。
考試合格後會收到來自Microsoft的作實電郵,並進入該電郵內的連結,登入 Microsoft Credentials Dashboard 下載您的證書。
考試不合格便可重新報考,不限次數。欲知道作答時間、題目總數、合格分數等詳細考試資料,可瀏覽本中心網頁 "各科考試分數資料"。 |
課程名稱:Microsoft Certified Azure AI Engineer Associate (1 科 Microsoft 雲端人工智能) 國際認可證書課程 - 簡稱:Azure AI Engineer Training Course |
AI-102 Designing and Implementing a Microsoft Azure AI Solution
Plan and manage an Azure AI solution
- Select the appropriate Azure AI service
- Select the appropriate service for a computer vision solution
- Select the appropriate service for a natural language processing solution
- Select the appropriate service for a speech solution
- Select the appropriate service for a generative AI solution
- Select the appropriate service for a document intelligence solution
- Select the appropriate service for a knowledge mining solution
- Plan, create and deploy an Azure AI service
- Plan for a solution that meets Responsible AI principles
- Create an Azure AI resource
- Determine a default endpoint for a service
- Integrate Azure AI services into a continuous integration and continuous delivery (CI/CD) pipeline
- Plan and implement a container deployment
- Manage, monitor, and secure an Azure AI service
- Configure diagnostic logging
- Monitor an Azure AI resource
- Manage costs for Azure AI services
- Manage account keys
- Protect account keys by using Azure Key Vault
- Manage authentication for an Azure AI Service resource
- Manage private communications
Implement content moderation solutions
- Create solutions for content delivery
- Implement a text moderation solution with Azure AI Content Safety
- Implement an image moderation solution with Azure AI Content Safety
Implement computer vision solutions
- Analyze images
- Select visual features to meet image processing requirements
- Detect objects in images and generate image tags
- Include image analysis features in an image processing request
- Interpret image processing responses
- Extract text from images using Azure AI Vision
- Convert handwritten text using Azure AI Vision
- Implement custom computer vision models by using Azure AI Vision
- Choose between image classification and object detection models
- Label images
- Train a custom image model, including image classification and object detection
- Evaluate custom vision model metrics
- Publish a custom vision model
- Consume a custom vision model
- Analyze videos
- Use Azure AI Video Indexer to extract insights from a video or live stream
- Use Azure AI Vision Spatial Analysis to detect presence and movement of people in video
Implement natural language processing solutions
- Analyze text by using Azure AI Language
- Extract key phrases
- Extract entities
- Determine sentiment of text
- Detect the language used in text
- Detect personally identifiable information (PII) in text
- Process speech by using Azure AI Speech
- Implement text-to-speech
- Implement speech-to-text
- Improve text-to-speech by using Speech Synthesis Markup Language (SSML)
- Implement custom speech solutions
- Implement intent recognition
- Implement keyword recognition
- Translate language
- Translate text and documents by using the Azure AI Translator service
- Implement custom translation, including training, improving, and publishing a custom model
- Translate speech-to-speech by using the Azure AI Speech service
- Translate speech-to-text by using the Azure AI Speech service
- Translate to multiple languages simultaneously
- Implement and manage a language understanding model by using Azure AI Language
- Create intents and add utterances
- Create entities
- Train, evaluate, deploy, and test a language understanding model
- Optimize a language understanding model
- Consume a language model from a client application
- Backup and recover language understanding models
- Create a custom question answering solution by using Azure AI Language
- Create a custom question answering project
- Add question-and-answer pairs manually
- Import sources
- Train and test a knowledge base
- Publish a knowledge base
- Create a multi-turn conversation
- Add alternate phrasing
- Add chit-chat to a knowledge base
- Export a knowledge base
- Create a multi-language question answering solution
Implement knowledge mining and document intelligence solutions
- Implement an Azure AI Search solution
- Provision an Azure AI Search resource
- Create data sources
- Create an index
- Define a skillset
- Implement custom skills and include them in a skillset
- Create and run an indexer
- Query an index, including syntax, sorting, filtering, and wildcards
- Manage Knowledge Store projections, including file, object, and table projections
- Implement an Azure AI Document Intelligence solution
- Provision a Document Intelligence resource
- Use prebuilt models to extract data from documents
- Implement a custom document intelligence model
- Train, test, and publish a custom document intelligence model
- Create a composed document intelligence model
- Implement a document intelligence model as a custom Azure AI Search skill
Implement generative AI solutions
- Use Azure OpenAI Service to generate content
- Provision an Azure OpenAI Service resource
- Select and deploy an Azure OpenAI model
- Submit prompts to generate natural language
- Submit prompts to generate code
- Use the DALL-E model to generate images
- Use Azure OpenAI APIs to submit prompts and receive responses
- Optimize generative AI
- Configure parameters to control generative behavior
- Apply prompt engineering techniques to improve responses
- Use your own data with an Azure OpenAI model
- Fine-tune an Azure OpenAI model
The course content above may change at any time without notice in order to better reflect the content of examination.
|
|